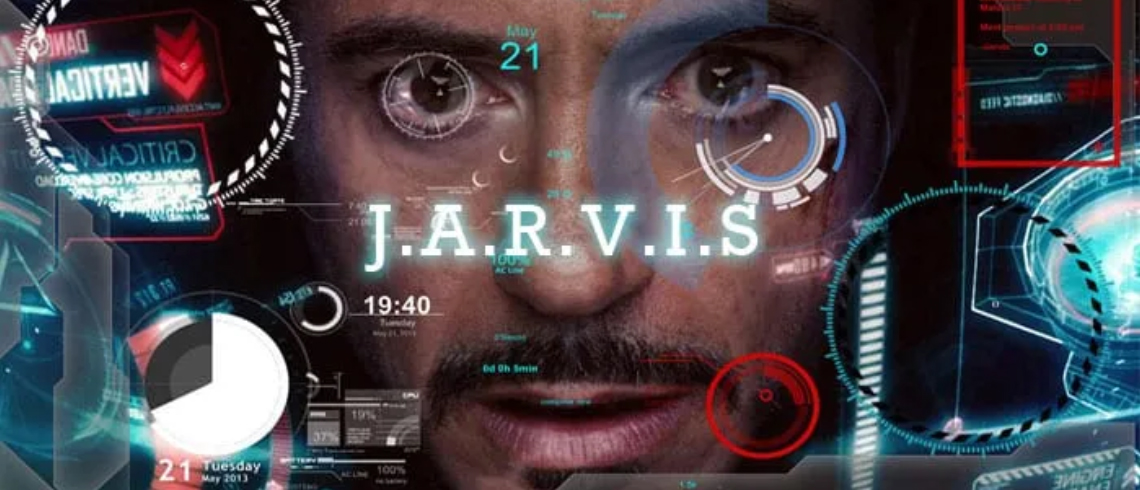
In today's world, we heavily rely on specialized AIs to solve complex problems such as content generation, image or voice recognition, and decision-making. However, for tasks such as video production, we often require a team of AIs, with a human captain to manage them. But what if we had a deputy to assist with the managerial work? Enter HuggingGPT, a system jointly developed by researchers from Zhejiang University and Microsoft Research Asia in Shanghai.
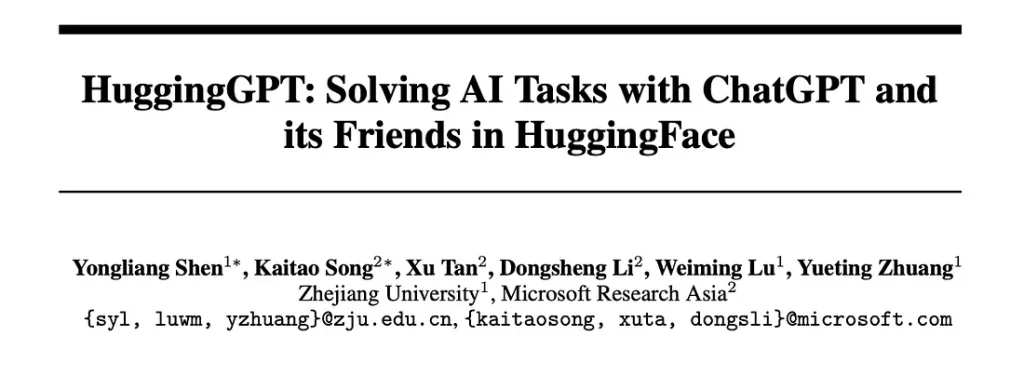
HuggingGPT uses large language models (LLMs) like ChatGPT to connect various AI models in machine learning communities (such as HuggingFace) to solve complicated AI tasks. It uses language as a generic interface to manage existing AI models and solve tasks in different domains and modalities, such as language, vision, speech, and others. By leveraging the strong language capability of ChatGPT and the abundant AI models in HuggingFace, HuggingGPT can cover numerous sophisticated AI tasks and achieve impressive results.
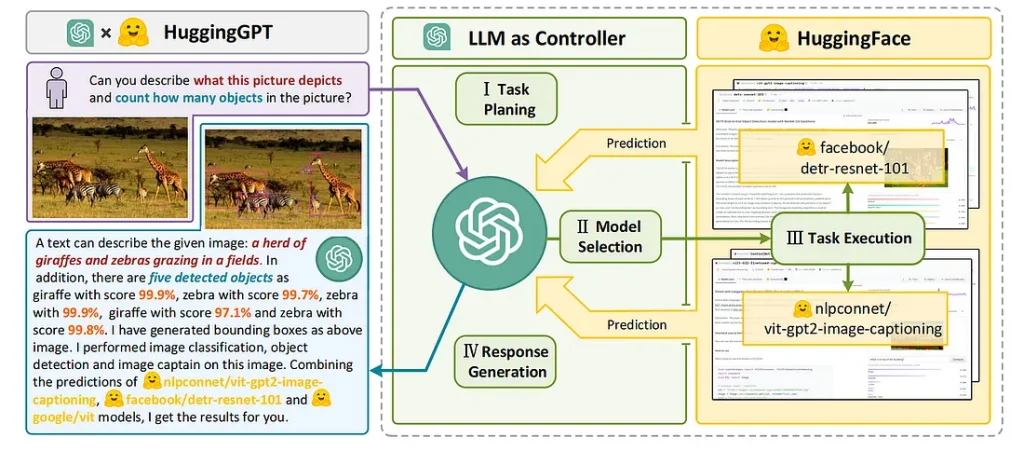
In the first stage of HuggingGPT, the large language model takes your big task and breaks it down into a bunch of smaller ones, figuring out which ones are most important and what order to do them in, just like a puzzle master. Then, HuggingGPT searches through a whole bunch of AI models to find the perfect ones for each of the tasks. For this purpose, the researchers first obtain the descriptions of expert models from the HuggingFace Hub and then dynamically select models for the tasks through the in-context task-model assignment mechanism.
Once it has everything it needs, it gets to work, i.e., to perform model inference. For speedup and computational stability, HuggingGPT runs these models on hybrid inference endpoints. Hybrid inference endpoints work by taking inputs from HuggingGPT, running them through a bunch of different programs, and then sending the results back to HuggingGPT to use. Think of it like a relay race: HuggingGPT hands off the baton to the hybrid inference endpoint, which runs with it for a while, and then hands the baton back to HuggingGPT when it’s finished. The result is that HuggingGPT can get things done faster and with more accuracy.
After all task executions are completed, HuggingGPT enters the response generation stage. In this stage, HuggingGPT integrates all the information from the previous three stages (task planning, model selection, and task execution) into a concise summary, including the list of planned tasks, the models selected for the tasks, and the inference results of the models.
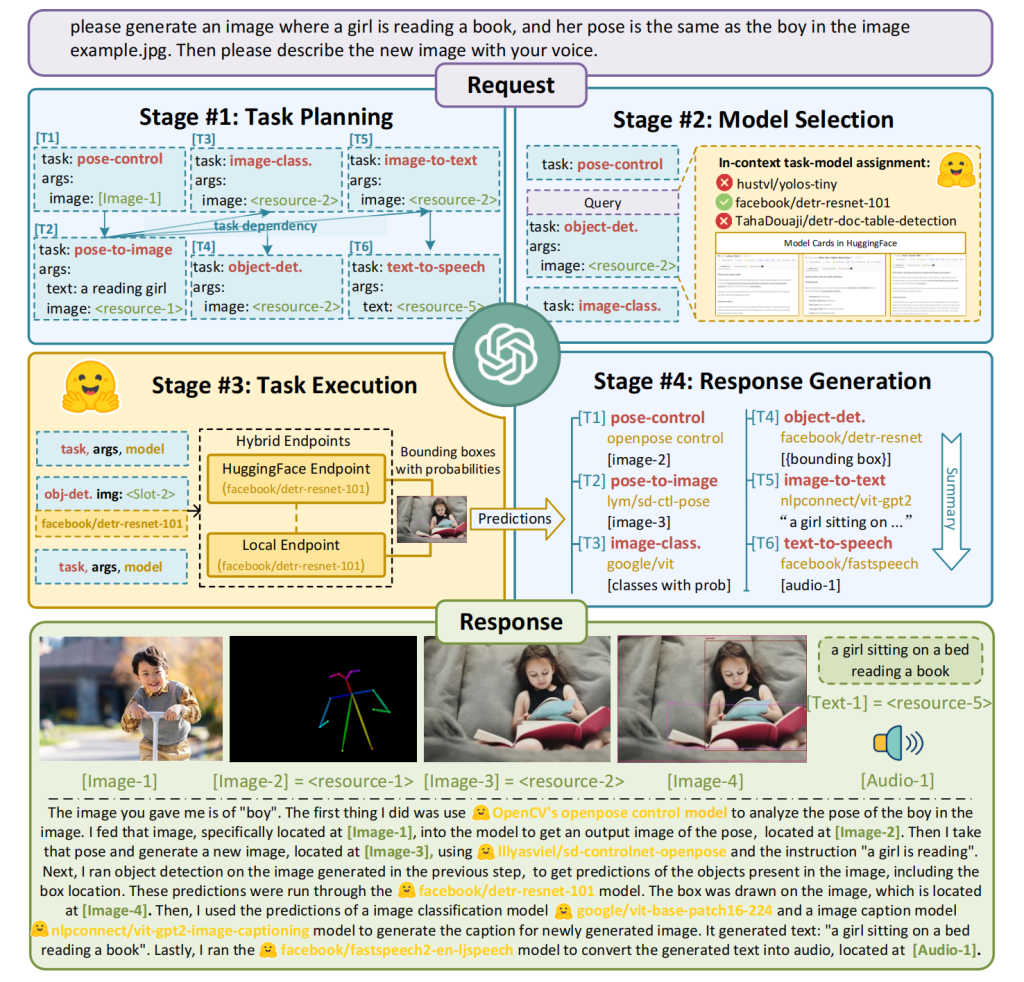
The researchers tested the ability of HuggingGPT with an image description task and found that HuggingGPT is good at understanding what you’re asking for, even if you don’t say it directly. It can take a simple request like “describe the image” and turn it into a series of smaller tasks like describing what’s in the image and answering questions about it. HuggingGPT knows which tools to use for each task and then puts all the information together to give you a detailed description. Sometimes, you might have a bunch of tasks in your request, but HuggingGPT can handle them all at once and give you answers for everything.
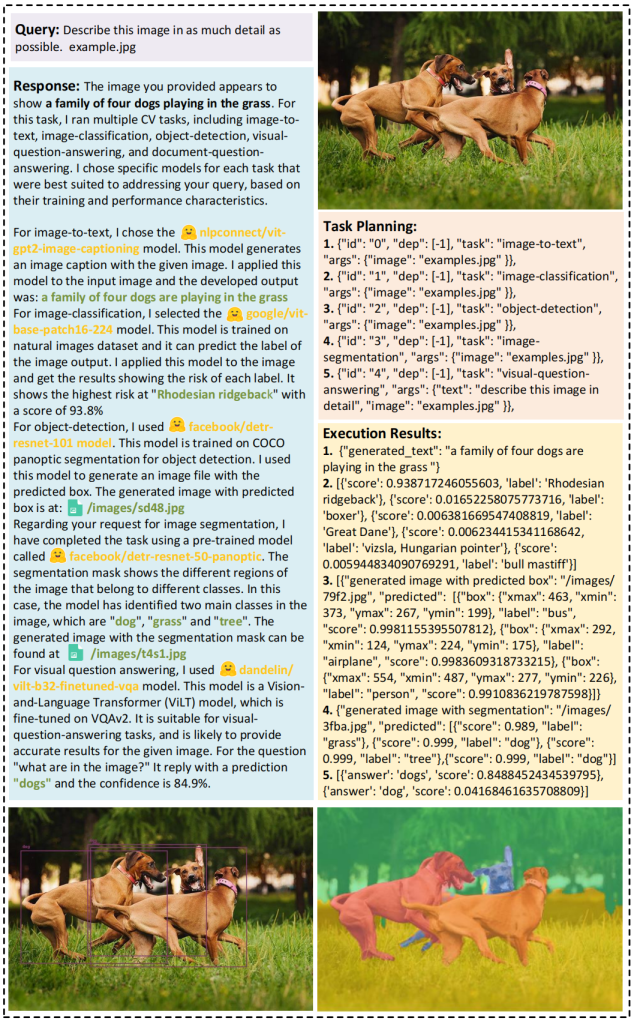
With the ability showcased here, in the near term, HuggingGPT might be able to automate a big chunk of the workflows for a lot of businesses. For the long term, the advent of AI collaborative systems like HuggingGPT paves the way for the long-anticipated artificial general intelligence.