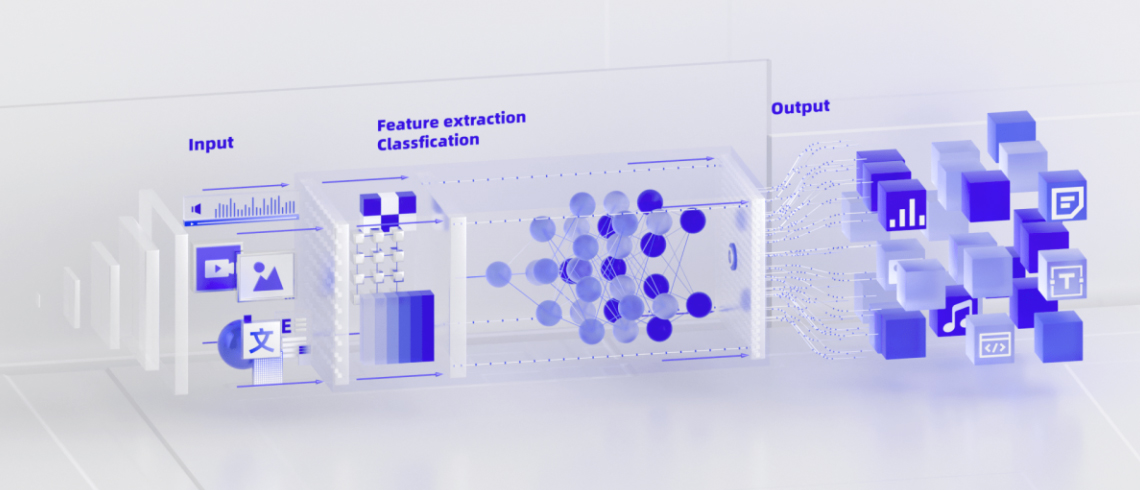
Pre-trained multimodal foundation models have become a new paradigm and infrastructure for building artificial intelligence (AI) systems. These models can acquire knowledge from different modalities and present the knowledge based on a unified representation learning framework.
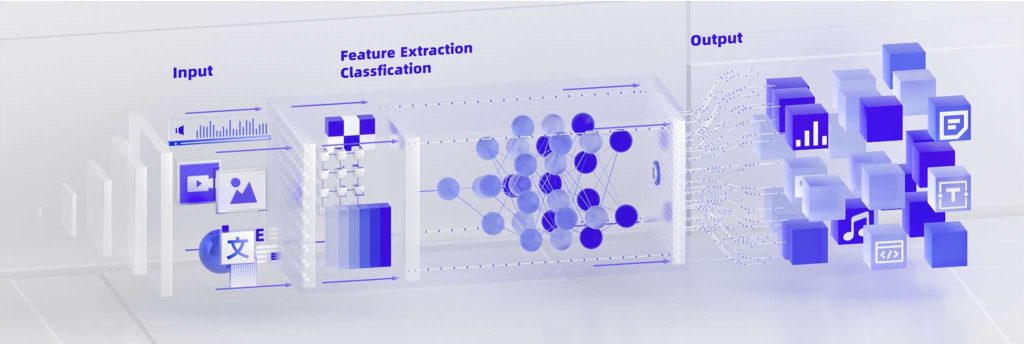
AI systems are evolving from mono-modal systems that focus on either text, speech, or visuals, towards general-purpose systems. At the core of these general-purpose systems is multimodality. Multimodality helps endow AI systems with cross-modal semantic enhancements, combine different modalities, and standardize AI models. The emergence of cutting-edge technologies, such as Contrastive Language-Image Pre-Training (CLIP) and the general-purpose multimodal foundation model BEiT-3, breathes new life into the development of AI. The future of AI is to develop multimodal foundation models that can transfer knowledge across tasks or scenarios based on a wide variety of intelligence accumulated from all industries. In the future, foundation models are set to serve as the basic infrastructure of AI systems across tasks of images, text, and audio, empowering AI systems with cognitive intelligence capabilities to reason, answer questions, summarize, and create.
The interconnect standards of chiplets will gradually converge into a unified standard, bringing in a new wave of change to the R&D process of integrated circuits (ICs).
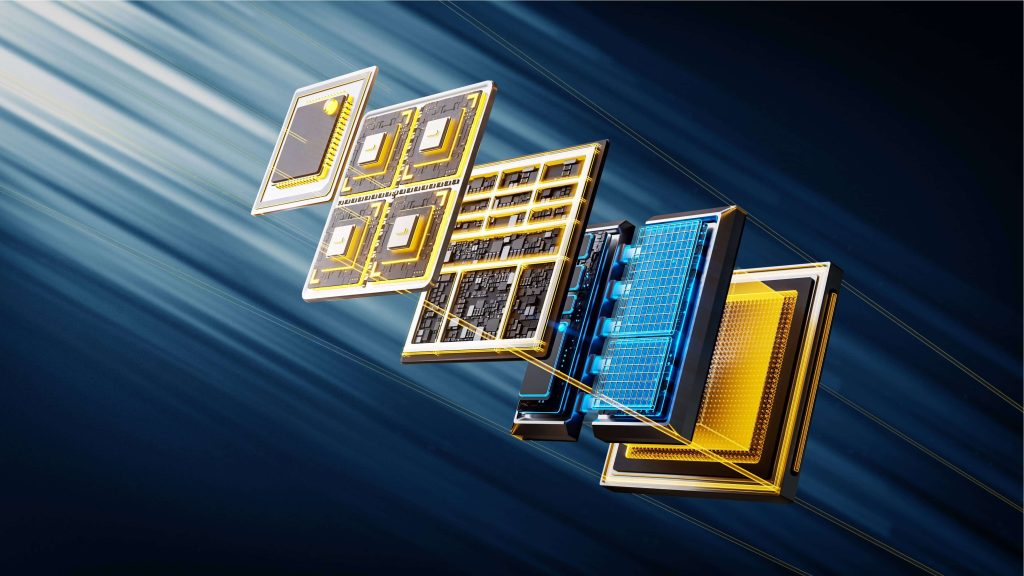
Chiplet-based design revolves around three core processes: deconstruction, reconstruction, and reuse. This method allows manufacturers to break down a System on a Chip (SoC) into multiple chiplets, manufacture the chiplets separately by using different processes, and finally integrate them into an SoC through interconnects and packaging. This method can significantly reduce costs and give rise to a new form of reusing intellectual property. As the integration and computing power of SoCs approach the physical limits, chiplets provide an important way to continuously improve the integration and computing power of SoCs. With the establishment of the Universal Chiplet Interconnect Express (UCIe) consortium in March 2022, the interconnect standards of chiplets are being unified into a single standard, accelerating the industrialization process of chiplets. Powered by advanced packaging technologies, chiplets may bring in a new wave of change to the R&D process of integrated circuits, from electronic design automation (EDA), design, and manufacturing to packaging and testing. This will reshape the landscape of the chip industry.
The large-scale commercial employment of compute-in-memory chips in vertical markets is mainly driven by the growing industrial demand and capital investment.
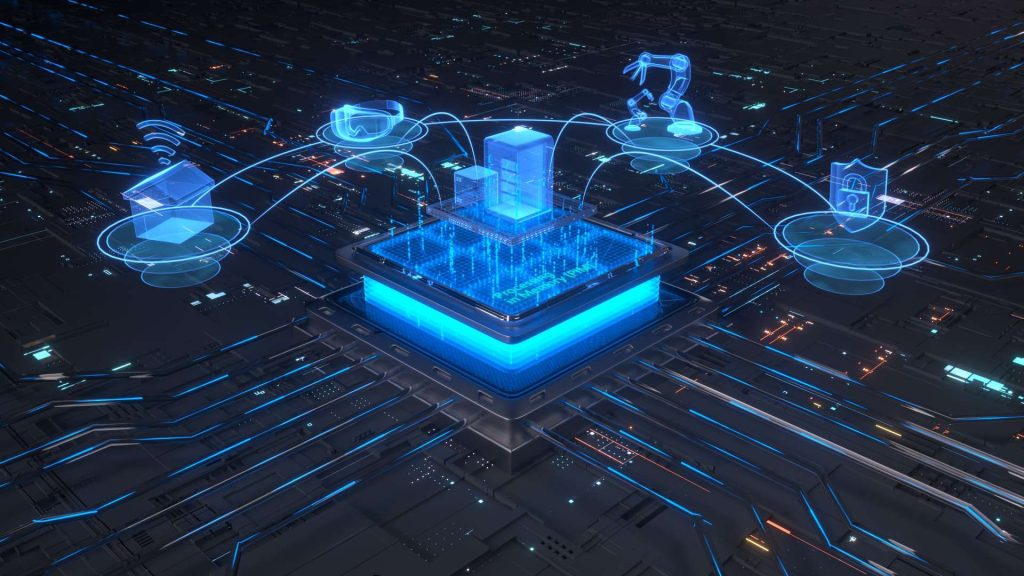
Processing in Memory (PIM) technology is the integration of a CPU and memory on a single chip, which allows data to be directly processed in memory. This not only reduces data transmission costs while improving computing performance, but also requires less energy to operate. PIM is an ideal solution for AI computing scenarios that require frequent, concurrent access to data. Driven by the growing industrial demand and capital investment, compute-in-memory chips are being rolled out from the production line and tested in real-world applications. These chips make use of static random-access memory (SRAM), dynamic RAM (DRAM), and Flash storage, and are targeted towards products that require low power consumption and low computing power, such as smart home appliances, wearable devices, robots, and smart security products. In the future, compute-in-memory chips are projected to be used in more powerful applications such as cloud-based inference. This will shift the traditional computing-centric architecture towards the data-centric architecture, which will have a positive impact on industries such as cloud computing, AI, and Internet of Things (IoT).
Security technologies and cloud computing become fully integrated, which boosts the development of new platform-oriented, intelligent security systems.
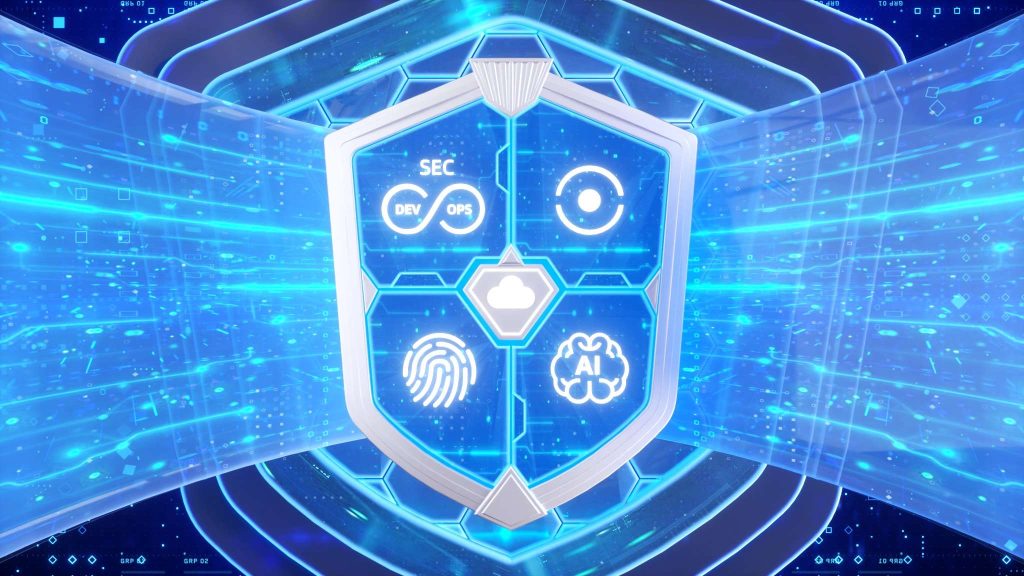
The approach of cloud-native security represents a shift from perimeter-focused defense to defense-in-depth, and from add-on deployment to built-in deployment. Cloud-native security is implemented to not only deliver security capabilities that are native to cloud infrastructure, but also improve security services by leveraging cloud-native technologies. As a result, security technologies and cloud computing are becoming more integrated than ever before. We have witnessed applied technologies evolve from containerized deployment to microservices and then to the serverless model, and security services embrace the shift to become native, fine-grained, platform-oriented, and intelligent.
- Native security services mean embracing the shift-left security approach to build a product security system. The system integrates product R&D, security, and O&M, boosting the collaboration among the R&D, security, and O&M teams.
- Fine-grained security services support precise access control and dynamic policy configuration while providing unified authentication and configuration management capabilities.
- Platform-oriented security services use a hierarchical in-depth defense system and a platform integrated with security products to implement precise proactive defense, eliminating the need to deploy a number of isolated security products.
- Intelligent security services are driven by security operations, and provide the following features to deliver end-to-end protection for applications, cloud products, and networks: real-time monitoring, precise response, quick attack tracing, and threat hunting.
Cloud computing is evolving towards a new architecture centered around CIPU. This software-defined, hardware-accelerated architecture helps accelerate cloud applications while maintaining high elasticity and agility for cloud application development.
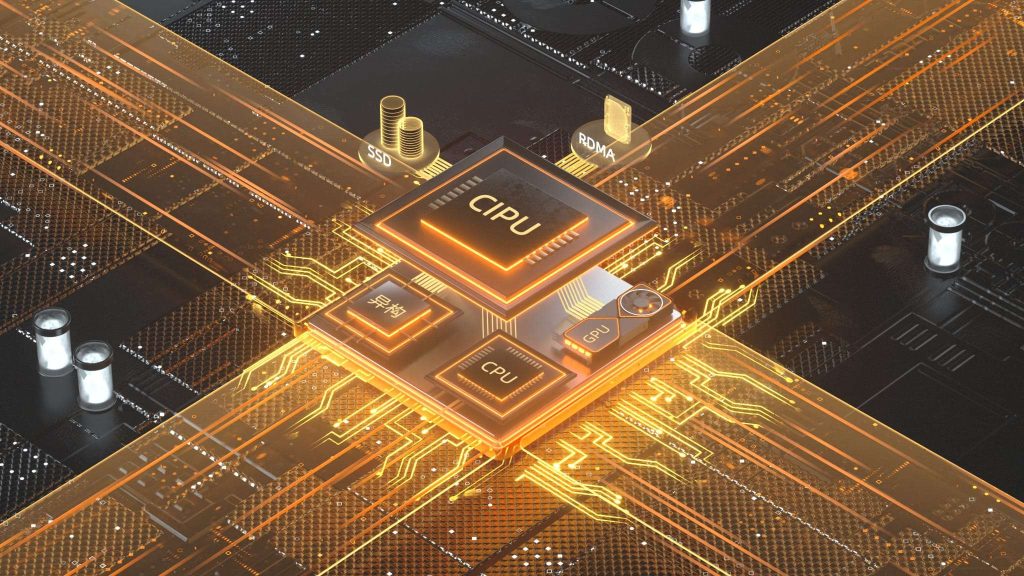
Cloud computing has evolved from the CPU-centric computing architecture to a new architecture centered around Cloud Infrastructure Processor (CIPU). This software-defined, hardware-accelerated architecture helps accelerate applications while maintaining high elasticity and agility for cloud application development. The new architecture incorporates both hardware infrastructure and software systems. As a processing unit, CIPU enables acceleration of hardware resources on the cloud, including computing, storage, and network resources. CIPU-accelerated hardware resources can connect to the distributed operating system through the cloud resource controller for flexible management, scheduling, and orchestration. CIPU will become the de facto standard of next-generation cloud computing and bring new development opportunities for core software R&D and dedicated chip design.
Cloud-defined predictable fabric featuring host-network co-design is gradually being adopted from data center networks to wide-area cloud backbone networks.
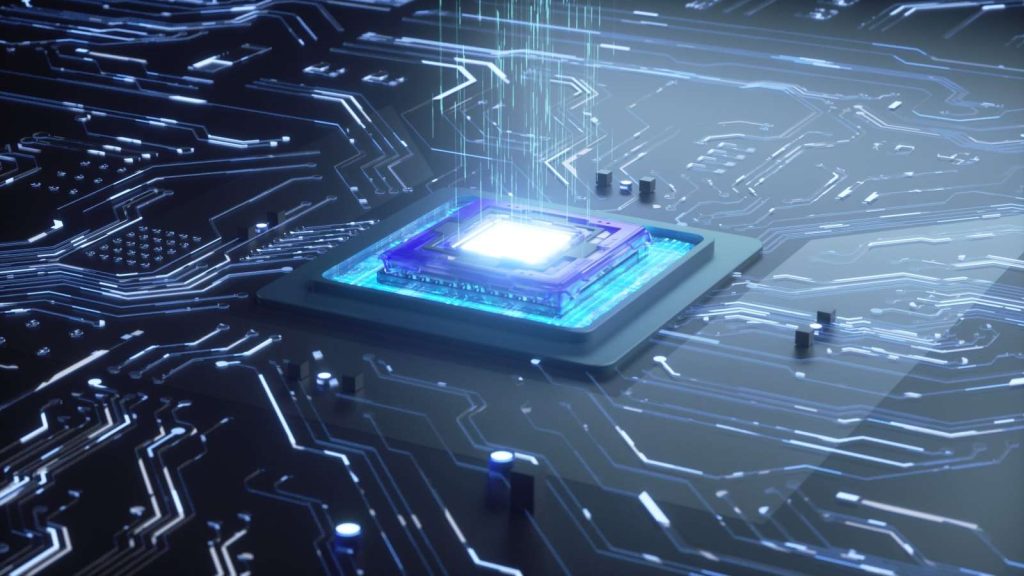
Predictable fabric is a host-network co-design networking system driven by advances in cloud computing, and aims to offer high-performance network services. It is also an inevitable trend as today’s computing and networking capabilities gradually converge on each other. On the cloud service provider’s side, predictable fabric paves the way for computing clusters to expand at scale through high-performance network capabilities, allowing the formation of large resource pools with immense computing power. On the consumer’s side, predictable fabric unlocks and delivers the full potential of computing power in large-scale industrial applications. Predictable fabric not only supports emerging large computing power and high-performance computing scenarios, but also applies to general computing scenarios. It represents the industrial trend of traditional network and future network integration. Through the full-stack innovation of cloud-defined protocols, software, chips, hardware, architecture, and platforms, predictable fabric is expected to subvert the traditional TCP-based network architecture and becomes part of the core network in next-generation data centers. Advances in this area are also driving the adoption of predictable fabric from data center networks to wide-area cloud backbone networks.
Decision intelligence supported by operations optimization and machine learning will facilitate dynamic and comprehensive resource allocation.
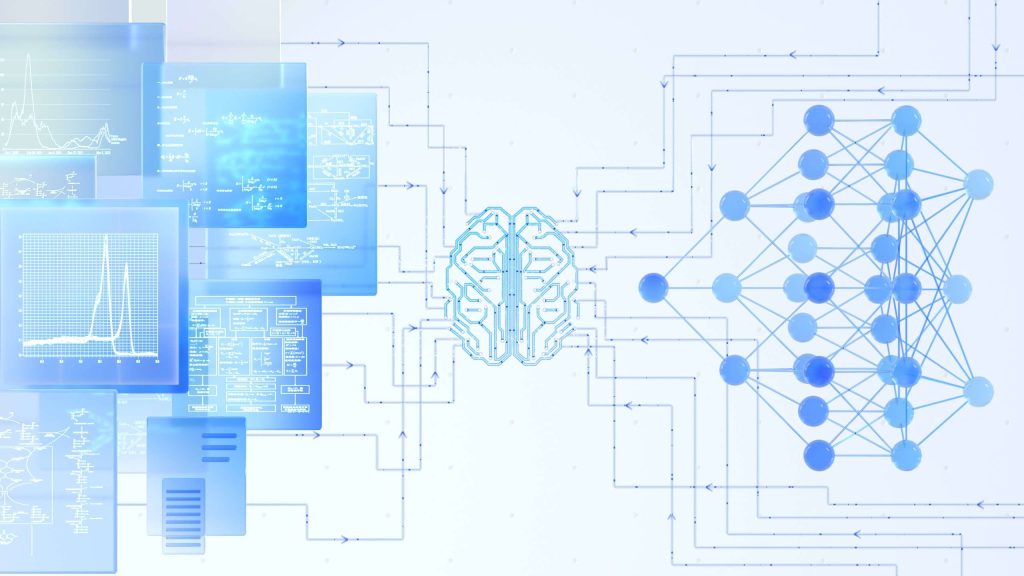
In today’s fast-changing world, enterprises must be able to take quick actions and make informed business decisions. The traditional decision-making method is based on Operations Research, which combines mathematical models built for real-life situations and algorithms for Operations Research optimization to find the best solutions under multiple constraints. However, as the world around us becomes more complex, this method is becoming less and less useful, due to its limitations in handling problems with great uncertainty and its slow response to large-scale problems. Therefore, academia and industry began to introduce machine learning into decision optimization, building dual-engine decision intelligence systems that utilize both mathematical and data-driven models. The two engines are perfect complements to each other. When used in tandem, they can improve the speed and quality of decision making. This technology is expected to be widely used in a variety of scenarios to support dynamic, comprehensive, and real-time resource allocation, such as real-time electricity dispatching, optimization of port throughput, assignment of airport stands, and improvement of manufacturing processes.
Computational imaging goes beyond the limits of traditional imaging, and brings about more innovative and imaginative applications in the future.
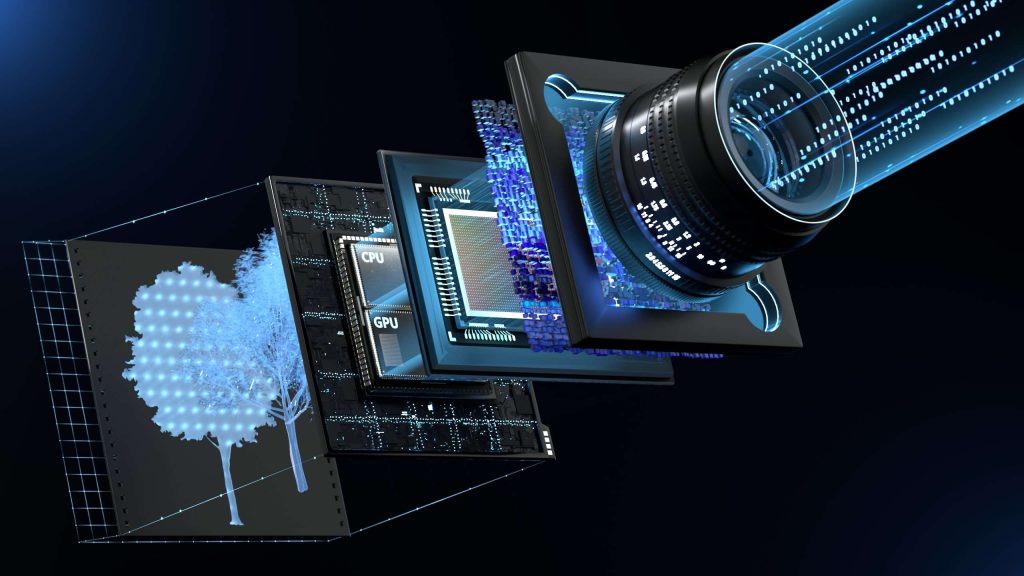
Computational imaging is an emerging interdisciplinary technology. This application-oriented technology collects and processes multidimensional optical information that cannot be detected by human eyes, such as lighting angles, polarization, and phases. It is a complete rethinking of the optical-based imaging system, and a new paradigm in the sensor technology. In contrast with traditional imaging techniques, computational imaging makes use of mathematical models and signal processing capabilities, and thus can perform unprecedented in-depth analysis on light field information. Computational imaging has been developing rapidly, with many promising research results. This technology has also been used on a large scale in areas such as mobile phone photography, health care, and autonomous driving. In the future, computational imaging will continue to revolutionize traditional imaging technologies, and bring about more innovative and imaginative applications such as lensless imaging and polarization imaging.
Large-scale urban digital twin technology is evolving towards becoming more autonomous and multidimensional.
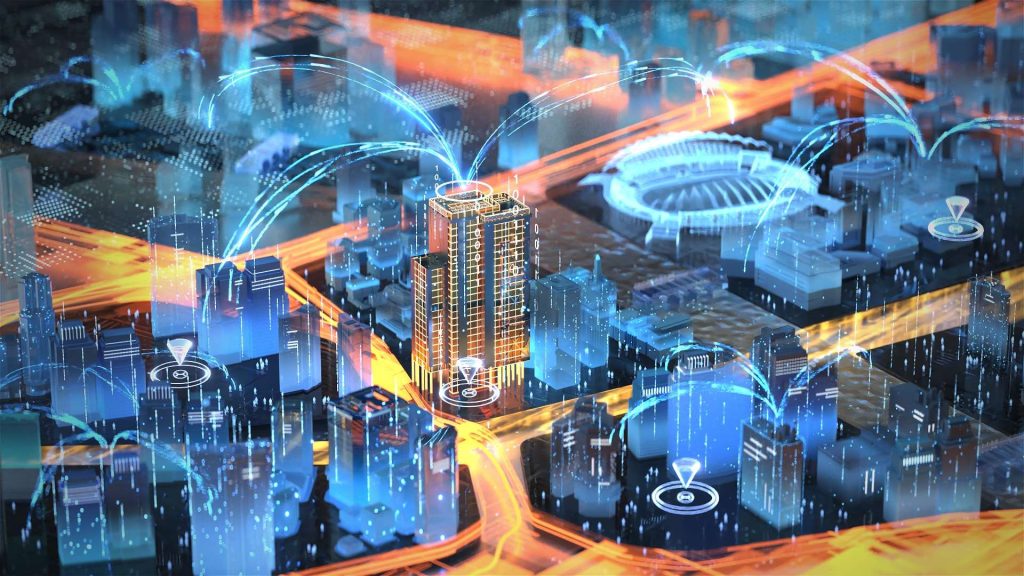
The concept of the urban digital twin has been widely promoted and accepted since its debut in 2017 and has become a new approach to refined city governance. In recent years, we have witnessed significant technical breakthroughs in this field. Several technologies are enhanced for large-scale application. For example, large-scale dynamic perceptual mapping lowers modeling costs, large-scale online real-time rendering shortens the response time, and large-scale joint simulation-enabled inference achieves higher precision. So far, large-scale urban digital twins have made major progress in scenarios such as traffic governance, natural disaster prevention, and carbon peaking and neutrality. In the future, large-scale urban digital twins will become more autonomous and multidimensional.
The widespread application of Generative AI is transforming how digital content is produced.
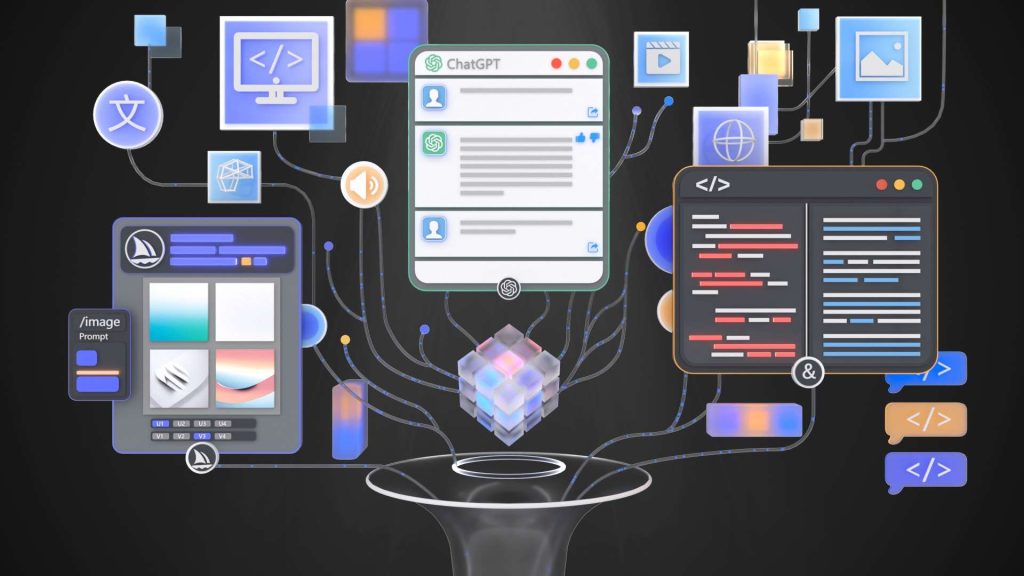
Generative AI (or AI-generated content, AIGC) generates new content based on a given set of text, images, or audio files. In 2022, its progress is most prominent in the following fields:
- image generation, driven by diffusion models (DALL·E-2 and Stable Diffusion)
- natural language processing (ChatGPT based on GPT-3.5)
- code generation (GitHub Copilot based on OpenAI Codex).
Currently, Generative AI is mainly used to produce prototypes and drafts and is applied in scenarios like gaming, advertising, and graphic design. Along with future technological advancement and cost reduction, Generative AI will become an inclusive technology that can greatly enhance the variety, creativity, and efficiency of content creation.